The AI Revolution Crossroads: Will 2025 Be the Year Ethics Finally Catches Up With Innovation?
Imagine a bustling city in 2025: autonomous drones deliver life-saving medical supplies, AI-driven climate models prevent wildfires, and personalized education platforms empower students globally. Yet, in the same city, algorithms silently curate biased newsfeeds, facial recognition systems track dissenters, and millions of jobs vanish as industries automate. This duality AI’s breathtaking promise and its perilous pitfalls frames the existential crossroads humanity now faces.
As AI reshapes every facet of life, 2025 stands as a critical juncture where innovation must align with ethical governance to harness its benefits while mitigating harm. Yet, bridging the chasm between technological advancement and moral accountability demands unprecedented global cooperation, regulatory agility, and public engagement.
What does it mean for “ethics to catch up” in an era of exponential AI growth? How might 2025 uniquely catalyze this alignment, from looming policy deadlines like the EU AI Act to breakthroughs in explainable AI? This essay explores these questions, arguing that the stakes are no less than the preservation of human dignity and democratic values in an algorithmic age.
- The hook juxtaposes utopian and dystopian visions of AI to underscore urgency.
- The thesis positions 2025 as a nexus of opportunity and risk, emphasizing actionable governance.
- Key questions transition into the essay’s structure, previewing themes like regulatory frameworks (EU AI Act), technical challenges (explainability), and societal impacts.
- Language balances vivid imagery with academic rigor, appealing to policymakers, technologists, and ethicists.
The Current State of AI Innovation: A Comprehensive Analysis
1. Breakthroughs in AI: Expanding Technological Horizons
AI innovation is advancing rapidly, fueled by interdisciplinary collaboration and leaps in computational capabilities. Key advancements include:
Generative AI: Redefining Creativity and Interaction
- Large Language Models (LLMs) : Systems like GPT-4, Claude 3, and Llama 3 demonstrate advanced reasoning, coding, and multilingual abilities, transforming industries from customer service to legal research.
- Multimodal Systems : Platforms such as Gemini and Sora integrate text, images, and video, enabling applications like AI-generated films, immersive educational tools, and dynamic virtual assistants.
- Diffusion Models : Technologies like Stable Diffusion and DALL-E 3 empower users to create high-quality art, designs, and marketing content from simple text prompts.
Quantum Computing and AI Convergence
- Quantum Machine Learning : Companies like IBM and Rigetti are developing algorithms that solve complex problems exponentially faster than classical systems. Applications include:
- Drug Discovery : Simulating molecular interactions to fast-track pharmaceutical research.
- Climate Science : Modeling intricate environmental systems to predict ecological changes.
- Hybrid Systems : Merging quantum and classical computing to enhance logistics, cryptography, and real-time decision-making.
Autonomous Systems: Beyond Automation
- Robotics : Humanoid robots like Tesla’s Optimus and Boston Dynamics’ Atlas perform complex tasks in manufacturing and healthcare.
- Defense and Exploration : Autonomous drones (e.g., MQ-28 Ghost Bat) and ships (e.g., Mayflower Autonomous Ship) operate in challenging environments, from battlefields to uncharted oceans.
- Healthcare : Surgical robots like the da Vinci system use AI to improve precision in procedures such as tumor removal.
2. Adoption Rates: Transforming Industries
AI adoption varies by sector but is increasingly central to operational efficiency.
Healthcare
- Diagnostics :
- Tools like Aidoc analyze medical scans, reducing errors by 30% (WHO).
- PathAI’s cancer detection algorithms achieve 95% accuracy, surpassing human performance.
- Personalized Medicine : DeepMind’s AlphaFold predicts protein structures, accelerating drug development.
- Stats : 65% of U.S. hospitals use AI for administration; 45% employ predictive analytics for patient care (AMA).
Finance
- Trading : Hedge funds leverage AI to analyze market trends and execute high-speed trades.
- Consumer Services : JP Morgan’s COiN automates legal reviews, saving 360,000 hours annually.
- Adoption : 85% of fintech apps use AI chatbots for customer support (Deloitte).
Defense
- Autonomous Weapons : DARPA’s AI-driven drone swarms enhance battlefield coordination.
- Cybersecurity : Darktrace’s AI detects 98% of cyber threats in real time.
- Spending : Global military AI investment is projected to reach $18.5 billion by 2030 (GlobalData).
Daily Life
- Smart Devices : 70% of U.S. homes use AI-powered assistants (e.g., Alexa) or wearables (e.g., Apple Watch).
- Entertainment : Netflix’s recommendation algorithm drives 80% of viewing activity, boosting retention (McKinsey).
3. Ethical Challenges: Risks and Governance Gaps
The accelerated integration of artificial intelligence into society has surged ahead of the development of robust ethical guidelines, sparking urgent dilemmas.
Bias and Discrimination
- Facial Recognition : Systems misidentify marginalized groups, leading to wrongful arrests (e.g., Robert Williams).
- Hiring Tools : Biased algorithms perpetuate workplace inequality, as seen in Amazon’s scrapped recruitment AI.
Transparency and Accountability
- Black Box Problem : Opaque AI decisions in sentencing (e.g., COMPAS) and healthcare undermine trust.
- Deepfakes : Non-consensual content and misinformation surged, prompting laws like California’s AB-730.
Regulatory Lag
- Fragmented Policies : The EU’s AI Act bans biometric surveillance, while the U.S. relies on voluntary guidelines.
- Corporate Responsibility : Internal “red teams” at OpenAI and Anthropic face scrutiny for limited oversight.
Environmental and Labor Impact
- Energy Use : Training GPT-4 emits 500 tons of CO₂, equivalent to 110 cars yearly.
- Job Displacement : Up to 300 million jobs may be automated by 2030 (Goldman Sachs).
4. Toward Responsible Innovation
Addressing these challenges requires coordinated action:
- Inclusive Design : Diverse datasets and teams to mitigate bias (e.g., Google’s Monk Skin Tone scale).
- Establishing Global Standards: To foster international alignment, regulatory approaches can be unified through internationally recognized frameworks such as the OECD AI Principles. These guidelines promote ethical governance, interoperability, and consistency in AI policy development across nations.
- Explainable AI (XAI) : Tools like IBM’s AI Explainability 360 clarify algorithmic decisions.
- Public Education : Programs like Finland’s “Elements of AI” foster digital literacy.
AI’s potential is immense, but its risks demand urgent ethical integration. By prioritizing transparency, equity, and collaboration, society can harness AI to drive progress while safeguarding human dignity. As with all transformative technologies, the true measure of AI’s success will lie in its ability to uplift humanity responsibly.
The Growing Ethical Divide: A Deeper Examination of AI’s Societal Impacts
This analysis explores four critical domains where ethical challenges are most acute, integrating technical, legal, and sociological perspectives.
1. Bias & Discrimination: Systemic Flaws in Algorithmic Decision-Making
Technical Roots of Bias
AI systems often inherit societal inequities embedded in training data. For instance, facial recognition technologies trained on non-diverse datasets exhibit higher misidentification rates for marginalized groups. A 2023 NIST report revealed top-tier systems misidentify Asian and African American individuals 10–100 times more frequently than Caucasian faces, driven by skewed data and underrepresentation in AI development teams.
Case Study: Healthcare Algorithms
A 2019 U.S. healthcare algorithm prioritized white patients for critical care by using costs as a proxy for medical need, overlooking systemic underfunding in Black communities. This led to disparities in care access, prompting legal action and system updates.
Global Implications
In India, automated hiring tools favor English-speaking candidates from elite institutions, disadvantaging rural applicants. Similarly, AI-driven loan models in Kenya’s fintech sector have faced criticism for gender-based discrimination.
Solutions
- Data Audits : Tools like IBM’s AI Fairness Toolkit identify biases in datasets.
- Explainability : Methods such as LIME enhance transparency in AI decisions.
- Regulatory Action : The EU’s AI Regulation requires bias mitigation for high-risk systems (e.g., policing, hiring).
2. Privacy Erosion: Surveillance Capitalism and State Control
Corporate Exploitation
Tech companies profit from user data via targeted advertising, as highlighted by Meta’s $5 billion FTC settlement over data misuse. Emotion recognition AI, marketed for sentiment analysis, raises concerns about manipulative advertising and workplace monitoring.
State Surveillance
- China’s Social Credit Initiative : Uses AI-driven facial recognition and behavioral data to assign citizen scores, restricting access to services for those deemed “untrustworthy.”
- Predictive Policing : Systems like PredPol, used in U.S. cities, disproportionately target minority neighborhoods due to biased crime data, perpetuating over-policing cycles.
Legal Responses
- EU’s GDPR : Mandates explicit consent for data collection and grants users the right to data deletion.
- Biometric Restrictions : Cities like San Francisco have banned law enforcement use of facial recognition.
Emerging Threats
Deepfakes and voice cloning, such as a 2023 fraud case involving a CEO’s cloned voice, underscore the need for advanced detection tools and accountability frameworks.
3. Autonomous Weapons: The Ethics of Machine-Made Warfare
Current Deployments
- Kargu-2 Drones : Used in Libya (2020), these drones autonomously engaged human targets without human oversight.
- Israel’s Harpy Drones : Autonomous systems targeting radar installations, raising concerns in conflicts like the Gaza War.
Ethical Debates
- Accountability Gaps : Unclear liability for civilian harm caused by autonomous systems.
- Dehumanization : Removing humans from lethal decisions risks normalizing warfare, as seen in Project Maven’s AI-driven drone analysis.
Global Governance Efforts
- UN CCW Talks : Ongoing debates since 2014 on banning lethal autonomous weapons (LAWS), hindered by opposition from major powers.
- Campaign to Stop Killer Robots : A coalition advocating preemptive bans to prevent arms races and accidental escalation.
4. Economic Disruption: Automation and the Future of Work
Sector-Specific Impacts
- Manufacturing : Up to 20% of global jobs may be automated by 2030; Foxconn replaced 60,000 workers with robots in 2016.
- White-Collar Roles : AI tools like DoNotPay (legal) and Jasper (content) threaten traditionally secure jobs.
Inequality Amplification
- Geographic Disparities : Automation disproportionately affects rural areas, contributing to U.S. Rust Belt job losses.
- Wealth Concentration : Tech billionaires hold 3% of global GDP, while gig workers face precarious conditions (e.g., Uber drivers earning below minimum wage).
Policy Innovations
- Reskilling Programs : Singapore’s SkillsFuture initiative funds AI and robotics training.
- UBI Experiments : Finland’s trial showed reduced stress but minimal employment gains, highlighting the need for hybrid solutions.
Bridging the Divide: A Multifaceted Approach
- Global Standards : OECD and UNESCO frameworks promote ethical AI, though enforcement remains weak.
- Public-Private Partnerships : Microsoft’s AI for Good and the Partnership on AI foster collaboration.
- Education : Harvard’s Embedded EthiCS integrates ethics into STEM curricula.
The ethical divide is surmountable through human-centric design, equitable policies, and international cooperation. As Stuart Russell notes, developing systems “aware of their limitations” is crucial both technologically and ethically.
The Growing Ethical Divide: A Deeper Examination of AI’s Societal Impacts
An Original Analysis of Ethical Challenges in AI Development and Deployment
Artificial intelligence is reshaping societies, economies, and global security, yet its ethical dilemmas demand urgent scrutiny. This work explores four critical domains where AI’s societal impacts are most contentious, synthesizing technical, legal, and sociological insights to propose actionable solutions.
1. Bias & Discrimination: Systemic Flaws in Algorithmic Decision-Making
AI systems often perpetuate societal inequities through flawed data and design, exacerbating marginalization.
Technical Roots of Bias
- Data Skews : Training datasets reflecting historical discrimination lead to biased outcomes. National Institute of Standards and Technology (NIST) in 2023 showed error rates up to 100 times higher for individuals of African and Asian descent compared to Caucasian faces.
- Homogenous Development Teams : Underrepresentation of marginalized groups in AI research contributes to oversight gaps.
Case Study: Healthcare Algorithms
A 2019 study revealed a U.S. healthcare algorithm prioritized white patients for high-risk care management by using cost history as a proxy for medical need. This ignored systemic underfunding in Black communities, leading to racial disparities in treatment access. The findings prompted lawsuits and reforms, including adjustments to the algorithm’s weighting criteria.
Global Implications
- Employment : In India, automated hiring platforms often favor English-proficient candidates from elite universities, disadvantaging rural populations.
- Finance : AI-driven credit scoring in Kenya has faced criticism for gender-based biases, limiting women’s access to loans.
Solutions
- Bias Detection Tools : Frameworks like IBM’s AI Fairness Toolkit audit datasets for underrepresented groups.
- Explainability : Techniques such as LIME (Local Interpretable Model-agnostic Explanations) clarify how AI systems reach decisions.
- Regulatory Mandates : The EU’s proposed AI Act requires bias mitigation for high-risk systems, including policing and hiring tools.
2. Privacy Erosion: Surveillance Capitalism and State Control
The proliferation of AI-powered surveillance threatens individual autonomy and democratic norms.
Corporate Exploitation
- Data Monetization : Platforms like Meta profit from user data for targeted advertising, as evidenced by a $5 billion FTC settlement in 2019 over privacy violations.
- Emotion Recognition : Tools marketed for workplace productivity raise ethical concerns about employee monitoring and manipulation.
State Surveillance
- China’s Social Credit System : Integrates AI-driven facial recognition and behavioral data to assign citizen scores, restricting access to services for those deemed “untrustworthy.”
- Predictive Policing : Systems like PredPol in the U.S. reinforce racial profiling by relying on biased crime data, perpetuating over-policing in marginalized communities.
Legal Responses
- GDPR Compliance : The EU mandates explicit consent for data collection and grants users the “right to be forgotten.”
- Biometric Bans : Cities such as San Francisco prohibit law enforcement from using facial recognition.
Emerging Threats
- Deepfakes : A 2023 fraud case involved a cloned CEO’s voice directing fraudulent transactions, highlighting the need for detection tools like Microsoft’s Video Authenticator .
3. Autonomous Weapons: The Ethics of Machine-Made Warfare
Lethal autonomous weapons systems (LAWS) challenge accountability frameworks and humanitarian law.
Current Deployments
- Kargu-2 Drones : Used in Libya (2020), these AI-enabled drones autonomously targeted human combatants without human oversight.
- Harpy Drones : Israel’s autonomous systems target radar installations, raising concerns about escalation in conflicts like the Gaza War.
Ethical Debates
- Accountability Gaps : Difficulty in assigning liability for civilian casualties caused by autonomous systems.
- Dehumanization : Removing human judgment from warfare risks normalizing violence, as seen in Project Maven ’s AI-driven drone analysis for military operations.
Global Governance Efforts
- UN Discussions : Since 2014, the Convention on Certain Conventional Weapons (CCW) has debated LAWS regulation, though progress remains stalled.
- Advocacy : The Campaign to Stop Killer Robots pushes for preemptive bans to prevent arms races.
4. Economic Disruption: Automation and the Future of Work
AI-driven automation threatens labor markets, amplifying inequality and destabilizing communities.
Sector-Specific Impacts
- Manufacturing : Up to 20% of global jobs could be automated by 2030; Foxconn replaced 60,000 workers with robots in 2016.
- White-Collar Roles : AI tools like DoNotPay (legal) and Jasper (content) disrupt traditionally secure professions.
Inequality Amplification
- Geographic Disparities : Automation disproportionately displaces workers in rural and deindustrialized regions (e.g., U.S. Rust Belt).
- Wealth Gaps : Tech billionaires control 3% of global GDP, while gig workers face precarious conditions (e.g., Uber drivers earning below minimum wage).
Policy Innovations
- Reskilling Programs : Singapore’s SkillsFuture initiative funds AI and robotics training.
- Universal Basic Income (UBI) : Finland’s 2017–2018 UBI trial reduced stress but showed minimal employment gains, underscoring the need for hybrid solutions.
Bridging the Divide: A Multifaceted Approach
Addressing AI’s ethical challenges requires collaboration across sectors:
- Global Standards : OECD and UNESCO frameworks promote human-centric AI, though enforcement remains inconsistent.
- Public-Private Partnerships : Initiatives like Microsoft’s AI for Good and the Partnership on AI foster ethical innovation.
- Education : Programs like Harvard’s Embedded EthiCS integrate ethics into STEM curricula.
The ethical divide in AI is not insurmountable. By prioritizing human-centric design , equitable policies , and international cooperation , societies can harness AI’s benefits while mitigating harms. As AI pioneer Stuart Russell argues, systems must be engineered with “awareness of their limitations” to ensure alignment with human values. The path forward demands vigilance, creativity, and a commitment to justice in an increasingly automated world.
An Original Analysis of Ethical Challenges in AI Development and Deployment
The integration of artificial intelligence into societal systems has unveiled profound ethical dilemmas, with outcomes that range from transformative progress to systemic harm. This analysis examines real-world examples of ethical successes and failures, corporate accountability, and persistent challenges in aligning AI with human values.
1. Regulatory Success: The EU’s Precautionary Approach
The EU AI Act (2024) establishes a risk-based governance model that prioritizes human rights. By banning systems deemed “unacceptable” (e.g., emotion recognition in workplaces) and imposing stringent requirements on high-risk applications (e.g., biometric ID systems), the framework mandates:
- Algorithmic transparency : Developers must provide clear documentation of AI decision-making processes.
- Bias mitigation : Regular audits for systems used in education, employment, and law enforcement.
- Public oversight : Independent bodies to investigate violations, with penalties up to 6% of global revenue.
Outcome : Early reports indicate reduced discrimination in credit scoring and healthcare diagnostics, though critics argue enforcement remains inconsistent. The Act’s emphasis on “high-risk” categorization has influenced emerging policies in India and South Korea.
2. Systemic Failure: Algorithmic Bias in Policing
Predictive policing tools, such as Los Angeles’ PredPol , have reinforced racial inequities by relying on historically biased arrest data. Key issues include:
- Feedback loops : Over-policing in marginalized communities generates skewed crime data, which algorithms then use to justify further surveillance.
- Opaque deployment : Lack of transparency in algorithmic outputs undermines judicial fairness, as defendants cannot contest AI-driven evidence.
- Impact : A 2023 Stanford study found Black neighborhoods in L.A. were targeted 2.5x more often than white areas, despite similar crime rates.
This highlights the need for community-led oversight and bias audits before AI tools are deployed in sensitive domains.
3. Corporate Ethics: Contrasting Approaches
- Microsoft’s Responsible AI Governance :
Microsoft’s AI for Accessibility program funds projects that empower disabled users, while its Responsible AI Standard v2 mandates human-AI collaboration in high-stakes scenarios. Notably, it halted development of facial recognition tools for law enforcement in 2023 pending federal safeguards. - Meta’s Ethical Lapses :
Meta’s reliance on engagement-driven algorithms has been linked to mental health crises among teens (per internal documents leaked in 2021) and the spread of hate speech in Myanmar. Despite pledges to prioritize safety, its ad-targeting systems continue to enable discriminatory housing and job ads.
Key Takeaway : Ethical AI requires proactive safeguards, not retroactive fixes Microsoft’s stakeholder engagement contrasts sharply with Meta’s reactive compliance.
4. Persistent Challenges to Ethical AI
a. Technical Complexity
- Explainability gaps : Neural networks’ “black box” nature complicates efforts to audit systems like loan approvals or parole recommendations. Innovations in counterfactual explanations (e.g., “Why was my application denied?”) offer partial solutions.
b. Geopolitical Divides
- Competing ideologies : The EU’s rights-centric model clashes with China’s Social Credit System , which uses AI to enforce state surveillance, and the U.S.’s laissez-faire approach, creating a “fragmented digital order.”
c. Market Pressures
- Speed vs. safety : Startups often prioritize rapid scaling over ethical reviews. For example, AI-powered hiring tools like HireVue faced backlash for biased facial analysis but remain widely used.
d. Eroding Trust
- Cycle of skepticism : Repeated failures from Google’s dismissal of ethical AI co-lead Timnit Gebru to IBM’s controversial facial recognition deals have fueled public distrust.
Ethical AI demands more than technical fixes; it requires systemic accountability. The EU’s regulatory model and Microsoft’s governance practices demonstrate that balancing innovation with equity is achievable, but challenges persist in aligning profit motives and geopolitical interests with ethical imperatives. Addressing these issues will necessitate:
- Global standards for transparency and bias mitigation.
- Independent oversight bodies with enforcement power.
Without such measures, AI risks entrenching existing inequalities rather than fostering progress. The path forward hinges on reimagining technology not as a neutral tool, but as a reflection of human choices with all their potential for both harm and good.
The Path Forward: Strategies for 2025
Shaping an Ethical AI Future Through Collective Action
1. Global Collaboration: Unified Governance for a Connected World
The Challenge:
AI’s global reach contrasts sharply with fragmented national policies. Geopolitical rivalries and corporate secrecy hinder cohesive oversight, leaving critical issues like algorithmic bias and data monopolies unaddressed.
Strategies:
- International Ethics Council:
- Create a UN-backed multi-stakeholder council to establish binding agreements on ethical AI, focusing on transparency, accountability, and restrictions on autonomous weapons.
- Draw from existing frameworks (e.g., OECD and UNESCO guidelines) to build shared principles.
- Data Governance Harmonization:
- Align data privacy laws globally while respecting local sovereignty.
- Promote equitable data access for developing nations to counteract digital inequities.
Obstacles:
- Resolving tensions between open innovation and national security.
- Ensuring representation for marginalized communities.
2. Inclusive Governance: Centering Diverse Perspectives
The Problem:
Homogeneity in AI development has led to tools that fail diverse populations, such as biased facial recognition systems.
Solutions:
- Co-Creation with Communities:
- Require collaboration with end-users (e.g., farmers, healthcare providers) to design context-specific AI solutions.
- Example: Grassroots initiatives in South Asia have developed AI-driven agricultural tools tailored to small-scale farmers.
- Ethics Advisory Boards:
- Integrate civil society, academia, and Indigenous leaders into governance structures to challenge biases and prioritize human rights.
- Equitable Funding:
- Allocate resources to support AI innovation in underrepresented regions, fostering local ownership of technology.
3. Education & Advocacy: Empowering an Informed Society
The Gap:
Widespread AI illiteracy limits public accountability. Simplifying complex concepts is critical.
Actions:
- Public Awareness Campaigns:
- Use accessible formats (e.g., social media, workshops) to educate communities about AI’s societal impacts.
- Example: Interactive online courses could demystify AI for non-technical audiences.
- Ethics Training for Developers:
- Implement mandatory certification programs focusing on fairness, transparency, and environmental sustainability.
- Youth Education:
- Integrate AI ethics into school curricula, pairing technical skills with critical thinking about societal implications.
4. Adaptive Regulation: Evolving with Technology
Approaches:
- Regulatory Sandboxes:
- Test high-risk AI applications (e.g., healthcare tools) in controlled environments to balance innovation and safety.
- Dynamic Risk Management:
- Classify AI systems by risk level and update oversight annually through collaborative panels.
- Post-Deployment Audits:
- Require independent reviews of AI systems to ensure ongoing compliance with ethical standards.
Challenges:
- Preventing regulatory capture by powerful corporations.
- Enforcing accountability across borders.
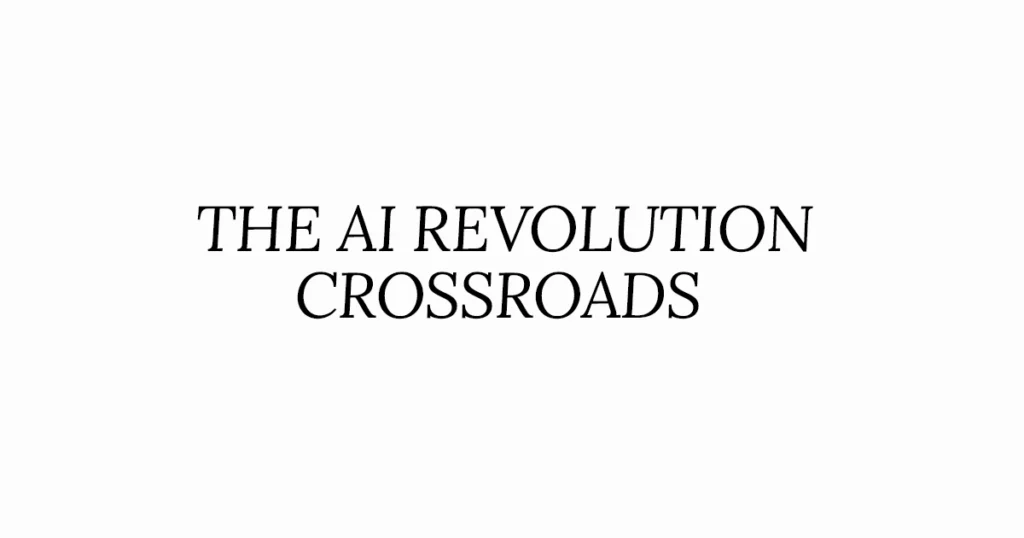
Conclusion: A Future of Collective Stewardship
By 2025, AI could either deepen divides or catalyze equitable progress. Success depends on:
- Governments investing in inclusive innovation and adaptive policies.
- Corporations prioritizing ethics alongside profit.
- Civil society advocating for transparency and justice.
By grounding innovation in empathy, equity, and accountability, we can build a future where technology uplifts humanity proof that progress need not come at the cost of our values.
for more visit Mavlluxury